I, Architect
IBM’s Watson was a winner on Jeopardy! Alexa and Siri are virtual assistants with conversational skills. Google Search knows what you are looking for, and Netflix knows what you should watch next. Driving apps find the fastest route home.
These examples of “artificial intelligence” are part of our daily lives, and more applications of AI and machine learning are on the horizon. These systems analyze data and images and then learn to make predictions to bring us better search results or more efficient driving routes. (These are powerful programs with advanced algorithms, but today’s “narrow” or “weak” AI can only simulate human cognition. “Strong” AI, with true intelligence and self-awareness, does not yet exist.)
Within the architectural/engineering/consulting industry, AI and machine learning is poised to impact the general design process as well as specific industries such as water resources and transportation.
An article by Kathleen O’Donnell for the American Institute of Architects highlighted the benefits of AI in the design industry, but noted that it will support – not replace – the creativity and problem-solving expertise of designers. “There are long-term benefits to investing time and resources into data and automation for every architect’s business,” O’Donnell wrote. “The opportunity to influence a new way to work is too big to ignore.”
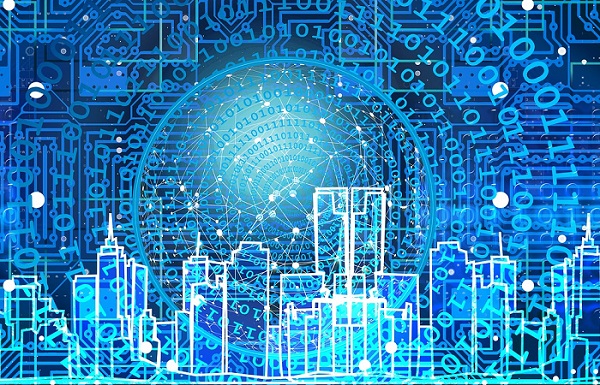
AI-driven solutions for the A/E/C industry are becoming more common, as the technology behind these innovations spread into nearly all aspects of our lives.
An article by Sumana Rao on the Trimble Constructible site offered 10 examples of how artificial intelligence can impact the construction industry. The possibilities range from using data to help manage cost overruns, risk, construction site safety, and how systems function after they are built or installed.
Data is plentiful within the construction industry, Rao noted, and more information will continue to flow from drones, cameras, and other sensors. “Every job site becomes a potential data source for AI,” Rao wrote. “…This presents an opportunity for construction industry professionals and customers to analyze and benefit from the insights generated from the data with the help of AI and machine learning systems.”
IMS is beginning to see a demand for consulting services to evaluate and implement AI/machine learning systems to support infrastructure projects. (IMS covers current RFPs on a daily basis and tracks future projects through Advance Notices for its A/E/C clients.)
One of the first AI-related projects that IMS covered, in September 2020, was to help prepare transportation agencies for this new era of data collection and analysis. The RFP, released by the Maine Department of Transportation, sought a consultant to conduct research on the traffic monitoring networks for the New England states and to prepare a pilot case study of using artificial intelligence techniques. The New England DOTs want to know what data is available now, what is coming, and how the data is collected, stored, shared, and analyzed (IMS 530980).
A more recent project shows how transportation departments are looking for specific applications for AI. The Missouri Department of Transportation issued an RFP in February 2022 to review and analyze data collection and analysis efforts performed by MoDOT staff and to recommend five work activities that could be replaced or augmented by artificial intelligence and machine learning (IMS 610403).
The Department is interested to learn how AI/ML can be used for services such as inventories and assessments of roadway assets, inspections, and design surveys. This is possible, in part, to improved data collection and high-resolution imagery.
“The use of AI and ML is an innovation that will continue to play a prominent role in transportation,” the MoDOT said. “The objective of this research is to provide MoDOT with tools and information to implement and leverage AI and ML to improve workforce and public safety, increase time efficiencies and operations, facilitate asset inventory, support maintenance decision making, and reduce overall costs to the agency.”
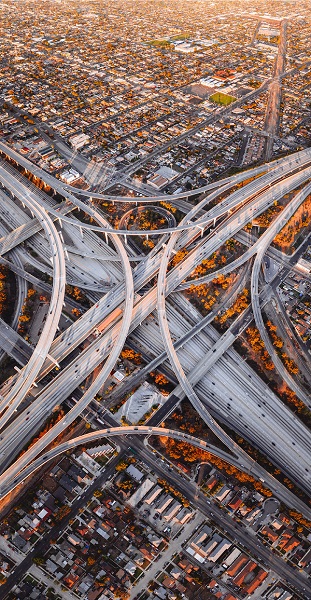
Data-heavy analytics in transportation and water management fields are prime examples of where machine learning can process information into novel results in a way that, in some cases, can exceed human ability. (Source: Denys Nevozhai)
This is not the Department’s first project to employ AI/ML. In response to a consultant’s question, the MoDOT said there were two earlier programs that leveraged machine learning.
Using machine learning, the KC Scout TMC and the Gateway Guide applications analyzed camera footage to identify “anomalous conditions” on the road. “They can detect things like wrong way drivers, stopped vehicles, pedestrians, and debris in the roadway,” the Department said. “The cameras have to be trained on the expected conditions, the location and number of the lanes, etc. After that they (the cameras) flag anything that does not match the expected conditions or breaks the rules.”
The second program featured the Waycare software system for the I-270 project. It’s another example of more data translated into more learning and better results. According to the MoDOT: “Included in the software is a crash prediction system that relies on a combination of historical data and live data streams. As operators confirm and dismiss the alerts and record the incidents, the system can improve its predictions.”
In another traffic-related project, the Virginia Department of Transportation is deploying an Artificial Intelligence-Based Decision Support System for the Northern Virginia and Fredericksburg area. The AI-DSS would analyze real-time data to understand current traffic conditions, and also to “predict future conditions” such as traffic jams, overcrowded bus and rail networks, and the availability of parking.
According to the Virginia DOT: “Under this AI-DSS procurement, VDOT is seeking an innovative system of solutions that (1) predict incidents, congestion, and parking availability; (2) select appropriate pre-agreed response plans to respond to actual and predicted events; and (3) furnish associated services to support these systems, including response plan development, response plan evaluation, alerts for agency actions, and an interface for agencies…”
Those are examples of state-led projects, but there is a desire to use AI/machine learning at the local level, too. The City of Arvada, Colo., released an RFP in February 2022 to demonstrate the costs and benefits of vendor software and hardware for traffic counting and surveillance AI systems (IMS 612792).
The City already collects data and imagery through its Miovision cameras and license plate readers. The challenge is how to review and analyze the hundreds of hours of video footage. Thus, the City seeks “AI to extract more information and leverage the power of computer analysis.”
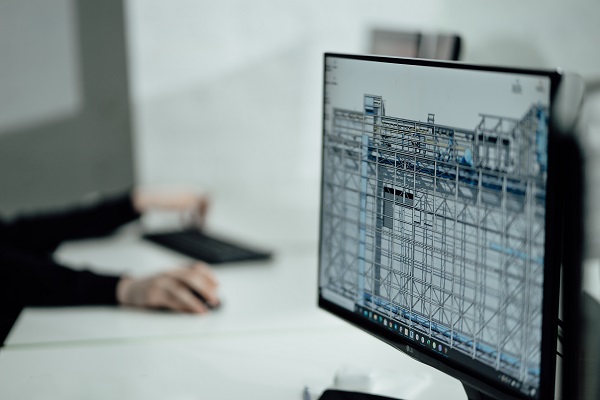
Computers and their associated technologies have long supported the A/E/C industry. The advent of AI/ML is a promising evolutionary step in this relationship.
The City is starting with a demonstration project at three locations, with the goal for the AI system to identify situations where drivers do not fully stop at an intersection, cars cross the centerline into the wrong lane, and where traffic lanes are blocked due to congestion.
AI/machine learning will also find a place in the water resources industry, where SCADA systems are scanning and collecting data every second. In December 2021, the City of Tacoma, Wash., released an RFI to help determine the feasibility of using artificial intelligence to optimize the performance of wastewater treatment plant operations (IMS 603223).
The AI system would be used alongside the current SCADA system, with its thousands of data points, to improve the effectiveness of the treatment process. The City is seeking AI to collect and analyze data, and then to deliver recommendations for the treatment plant.
According to the City, the AI software would “Detect real time changes in effluent and propose adjustments to improve effluent water quality” and also “Propose operational adjustments to reduce operating costs (i.e., reductions in energy or chemical usage).”
The A/E/C industry and public agencies have always collected massive amounts of data on infrastructure and construction projects. Now they are asking, How can machine learning programs and AI use that data to help with analysis, predictions, optimization, anticipation, and automation? It may look like this: Analyzing thousands of images to improve roadway safety. Predicting the failure of a pumping station or a structural bridge collapse due to weather and load conditions. Assessing and optimizing a water treatment process. Anticipating a traffic jam and re-routing traffic. It may even play a larger role automating elements of building design.